The goal of Logistic Regression
Suppose we have data with
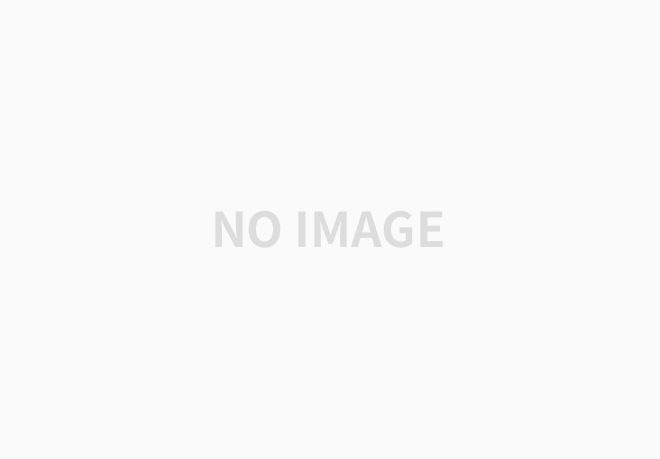
where:
is a scalar, representing the value of the -th feature for the -th sample (entry view). is a row vector, representing the values of all features for the -th sample (row view). is a column vector, representing the values of the -th feature for all samples (column view).
The goal of logistic regression is to classify the
The class label is denoted as:
where
indicates that the -th sample belongs to the positive class. indicates that the -th samtmple belongs to the negative class.
Class Probability
The logistic regression model does not directly predict
where:
and are parameters of the logistic regression model, optimized during the learning process. represents the weight for the -th feature; is the model’s bias term.
is the logit for the -th sample, also called the log-odds, and can be expressed as:
Logistic Function
The logistic function
It ensures that the output of model is always between 0 and 1, aligning with the probabilistic interpretation.
- If
, then . - If
, then . - If
, then .
By substituting the logit
- If
, then the -th sample is classified as belonging to the positive class ( ). - If
, then the -th sample is classified as belonging to the negative class ( ).
Cross-entropy Loss
In the logistic regression model, the cross-entropy loss (also known as the log-loss) is used to optimize the model parameters.
For a single sample
For
Here:
is the true label ( or ). is the predicted probability.
The parameters
Why Cross-entropy?
Information Theory Perspective
In information theory, the cross-entropy
For discrete data, it is defined as:
Here:
(true label, either or ). (predictied probability).
Logistic regression predicts the probability
Statistical Perspective
From a statistical perspective, logistic regression uses maximum likelihood estimation (MLE) to estimate the model parameters
The observed labels
The log-likelihood for a single observation is:
Thus, minimizing the cross-entropy loss is equivalent to maximizing the likelihood of the observed label.
'Mathematics' 카테고리의 다른 글
음이항분포 (기하분포)의 2가지 관점 (0) | 2025.02.24 |
---|---|
이벤트 발생 횟수와 대기 시간의 확률 모델들 (이항분포, 음이항분포, 푸아송 분포, 얼랑 분포) (0) | 2025.02.22 |
혼동행렬, 신호탐지이론, ROC 곡선 (0) | 2023.03.14 |
검정력, 적당한 표본의 크기 (0) | 2023.03.13 |
제1종 오류와 제2종 오류 (0) | 2023.03.12 |